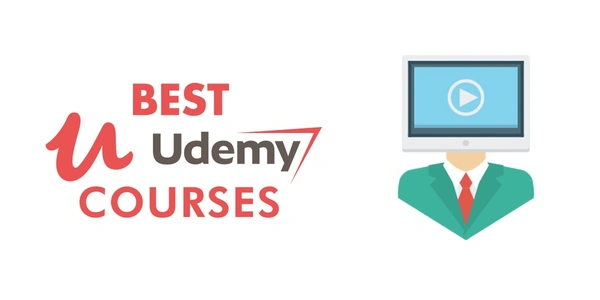
Free Download Udemy - XAI Explainable AI with InterpretML - Notebooks - Python
Published 4/2025
Created by Kishan Tongrao
MP4 | Video: h264, 1280x720 | Audio: AAC, 44.1 KHz, 2 Ch
Level: All | Genre: eLearning | Language: English | Duration: 37 Lectures ( 4h 54m ) | Size: 2.13 GB
Harnessing Explainable AI with InterpretML: Key Techniques in Model Interpretation, Feature Importance
What you'll learn
XAI Explainable AI
InterpretML Microsoft Library to do XAI
Linear Regression, Logistic Regression, APLR, Decision Tree, EBR, Random Forest, Shap Kernel, Lime Tabular, Partial Dependence, Morries Sensitivity Method
Shap Tree
Requirements
Basics of Python and Data Science
Description
Dive into the world of Explainable AI (XAI) with this comprehensive course, "XAI Explainable AI with InterpretML | Notebooks | Python." Designed for data enthusiasts and practitioners, this course introduces the fundamentals of XAI, emphasizing the critical importance of transparency and interpretability in machine learning models. Our key objectives include equipping you with practical skills to demystify complex models and enhance decision-making processes effectively.Through hands-on examples, you'll explore real-world applications of XAI using Python in Google Colab, with step-by-step guidance on installing and leveraging InterpretML. The course covers a wide range of techniques, starting with Linear Models and advancing to Additive Poisson Linear Regression (APLR) and Tree-based Models. You'll master powerful interpretability tools such as Explainable Boosting Regression (EBR), ShapKernel, and LimeTabular for deep tabular data insights. Additionally, we'll delve into Partial Dependence Plots, Morris Sensitivity Method, and SHAP Tree for robust feature analysis and comprehensive model behavior understanding.By the end, you'll be proficient in interpreting model predictions, identifying feature importance, and ensuring transparency in AI systems. Whether you're a beginner or an experienced data scientist, this course provides the practical tools and advanced techniques to make AI explainable, actionable, and trustworthy using InterpretML in Python. Join us to unlock the transformative power of XAI!
Who this course is for
Who wants to learn XAI Explainable AI using InterpretML Library
Homepage
Code:
Bitte
Anmelden
oder
Registrieren
um Code Inhalt zu sehen!
Recommend Download Link Hight Speed | Please Say Thanks Keep Topic Live
Code:
Bitte
Anmelden
oder
Registrieren
um Code Inhalt zu sehen!