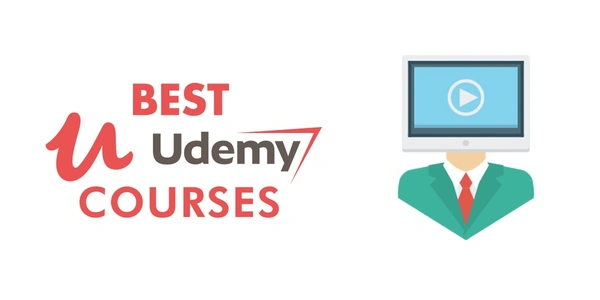
Free Download Udemy - Time-Series Analysis & Regression Forecasting with Python
Published 4/2025
Created by eTech School
MP4 | Video: h264, 1280x720 | Audio: AAC, 44.1 KHz, 2 Ch
Level: All | Genre: eLearning | Language: English | Duration: 71 Lectures ( 5h 45m ) | Size: 2 GB
Transform raw data into powerful forecasts with Python-learn time-series modeling, regression, real-world forecasting.
What you'll learn
Time-series concepts, notations, and use cases. Data loading, preprocessing, and feature engineering using Python.
Visualizing time-series data for insights. Forecasting using AR, MA, ARIMA, and SARIMA models.
Handling seasonality and trend components in data. Performing train-test splits and model validation correctly.
Applying linear regression (simple and multiple) for forecasting. Interpreting regression outputs and evaluating model accuracy.
Requirements
Basic knowledge of Python (variables, loops, basic functions).
Familiarity with basic statistics (mean, median, correlation).
Some exposure to pandas, matplotlib, or NumPy is helpful but not mandatory.
No prior experience with time-series or regression needed.
Description
Course Introduction:Forecasting is at the heart of modern data science, powering decision-making across finance, retail, healthcare, and beyond. This comprehensive course is your step-by-step guide to mastering time-series analysis and regression-based forecasting using Python. Whether you're a budding data scientist or an analyst aiming to add predictive analytics to your skillset, this course covers everything from basic notations to advanced models like ARIMA and SARIMA. You'll also learn how to prepare data for machine learning, visualize trends, and validate models like a pro.Through hands-on coding in Python, real-world use cases, and expert-led instruction, you'll gain the confidence to build and deploy forecasting models that actually drive impact.Section 1: Foundations of Time-Series Analysis in PythonStart your journey by understanding the fundamentals of time-series data-what makes it unique and why it matters in data science. You'll set up your environment with Anaconda and Jupyter, then dive into data loading, preprocessing, and feature engineering. You'll also learn how to visualize time-dependent patterns, apply transformations, and use basic statistical techniques like moving averages and exponential smoothing. By the end of this section, you'll be well-prepared for building time-aware models.Section 2: Time-Series Forecasting ModelsIn this section, you'll move from theory to practice with key time-series forecasting models. Starting with naive models, you'll progress to Auto-Regression (AR), Moving Average (MA), and ARIMA. Learn how to split time-series data properly, validate predictions using walk-forward validation, and interpret autocorrelation using ACF and PACF plots. You'll wrap up with SARIMA, an advanced seasonal model, and apply it all in Python through hands-on coding.Section 3: Data Preprocessing for Linear RegressionBefore building regression models, you need clean and meaningful data. This section teaches you the essential preprocessing steps required for high-quality regression modeling. You'll work through exploratory data analysis, outlier detection, missing value imputation, seasonality handling, and correlation analysis. You'll also transform variables, create dummy variables, and prepare your dataset for modeling. Each concept is reinforced with Python demos to solidify your understanding.Section 4: Building & Evaluating Regression ModelsFinally, bring your data to life by building regression models in Python. You'll understand how to apply the Ordinary Least Squares (OLS) method, interpret coefficients, and evaluate model performance using R-Squared, F-statistics, and more. You'll build both simple and multiple linear regression models, including handling categorical variables. This section ensures you're confident not only in model creation but also in explaining the results effectively.Course Conclusion:Congratulations! You've now developed the core skills required to perform both time-series forecasting and regression modeling using Python. From building clean datasets to visualizing trends and predicting future values, you're ready to tackle real-world forecasting challenges in any domain. Your ability to transform data into insight will set you apart as a capable and confident data professional.
Who this course is for
Aspiring data scientists and analysts looking to master forecasting.
Business intelligence professionals wanting to enhance reporting with prediction.
Students in data science or machine learning programs.
Python developers eager to apply coding skills to statistical forecasting.
Anyone curious about time-series analysis, regression, or predictive modeling.
Homepage
Code:
Bitte
Anmelden
oder
Registrieren
um Code Inhalt zu sehen!
Recommend Download Link Hight Speed | Please Say Thanks Keep Topic Live
Code:
Bitte
Anmelden
oder
Registrieren
um Code Inhalt zu sehen!