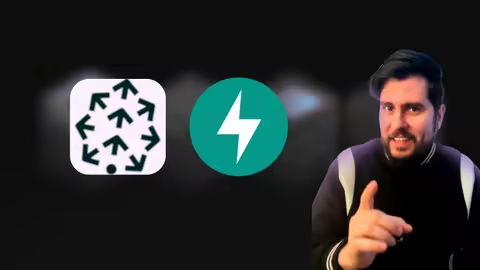
Free Download Udemy - Semantic Search With Vector Databases
Published: 3/2025
MP4 | Video: h264, 1920x1080 | Audio: AAC, 44.1 KHz
Language: English | Size: 4.48 GB | Duration: 5h 3m
From theory to practical implementation with Pinecone and AI
What you'll learn
Understand the fundamentals of semantic search and vector databases
Create and manage vector indexes with Pinecone
Implement text processing pipelines for vector databases
Develop advanced queries and AI assistants for vector search
Requirements
Basic programming skills (preferably in Python).
Introductory knowledge of APIs and HTTP requests.
Computer with internet access to test the codes in practice.
Free Pinecone account.
No previous experience with vector databases or semantic search is required.
Description
Semantic search and vector databases are transforming the way we deal with large volumes of information, making searches more precise and contextualized. If you want to understand this innovative technology and apply it to your projects, this course is for you.In this course, you will learn everything from the fundamental concepts of semantic search to the practical implementation of vector databases using Pinecone, one of the leading platforms for vector storage and retrieval. We will explore how to transform text into vectors, create efficient indexes and build intelligent queries to find relevant information quickly.What will you learn?Fundamentals of Semantic Search and Vector Databases - Understand how vectors represent meaning and context in searches.Creating and Managing Vector Indexes - Configure and manipulate vector databases using Pinecone.Text Processing for Embeddings - Extract data from documents, strategically split text, and generate high-quality embeddings.Building Advanced Query - Learn how to retrieve information efficiently and accurately.Developing an AI Assistant - Create a system that answers questions based on vector search.Who is this course for?This course is ideal for developers, data scientists, AI professionals, and students who want to deepen their knowledge of semantic search and vector databases. Whether you want to build recommendation systems, search engines, or intelligent chatbots, this course will provide you with the knowledge you need.No prior experience with vector databases is required.
Overview
Section 1: Module 1
Lecture 1 Introduction to Semantic Search and Vector Databases
Lecture 2 Creating your Pinecone account
Lecture 3 Capacity and vectors
Section 2: Module 2
Lecture 4 Introduction to creating indexes by code
Lecture 5 Setting up the environment and getting started
Lecture 6 Creating APIs for Pinecone
Lecture 7 Implementing index creation in Pinecone
Lecture 8 Integration with FastAPI and Creating Services
Lecture 9 Listing Indexes in Pinecone
Lecture 10 Displaying index details
Section 3: Module 3
Lecture 11 Introduction to Vector Index Manipulation
Lecture 12 Creating Embed Services to Transform Texts into Vectors Summary
Lecture 13 Extração de Texto de PDFs para Bancos Vetoriais
Lecture 14 Dividing Documents for Processing in Vector Databases
Lecture 15 Advanced Split Techniques with Overlap
Lecture 16 Implementing Split Functions in Code
Lecture 17 Processing Strategies for Embeddings
Section 4: Module 4
Lecture 18 Introduction to Upsert in Vector Databases
Lecture 19 Automating Upsert with Functions Customized
Lecture 20 Validating Insertions in Vector Databases
Lecture 21 Adding Metadata to Vectors in Pinecone
Lecture 22 Assembling Vectors with Metadata and Embeddings
Lecture 23 Validating and Testing Metadata in Pinecone
Section 5: Module 5
Lecture 24 Introduction to Queries in Vector Databases
Lecture 25 Building Queries in Vector Databases
Lecture 26 Formatting Responses to Vector Queries
Lecture 27 Creating an AI Assistant for Querying
Developers and data scientists who want to learn about semantic search and vector databases.,AI and Machine Learning professionals interested in optimizing information retrieval using embeddings.,Students and researchers working in natural language processing (NLP) who need to store and query large volumes of data efficiently.,Entrepreneurs and technology enthusiasts who want to build intelligent assistants, chatbots, and personalized search engines.
Homepage:
Code:
Bitte
Anmelden
oder
Registrieren
um Code Inhalt zu sehen!
Recommend Download Link Hight Speed | Please Say Thanks Keep Topic Live
Code:
Bitte
Anmelden
oder
Registrieren
um Code Inhalt zu sehen!